Scroll down for more
From Data to Action: Leveraging Predictive Analytics to Improve Supply Chain Performance
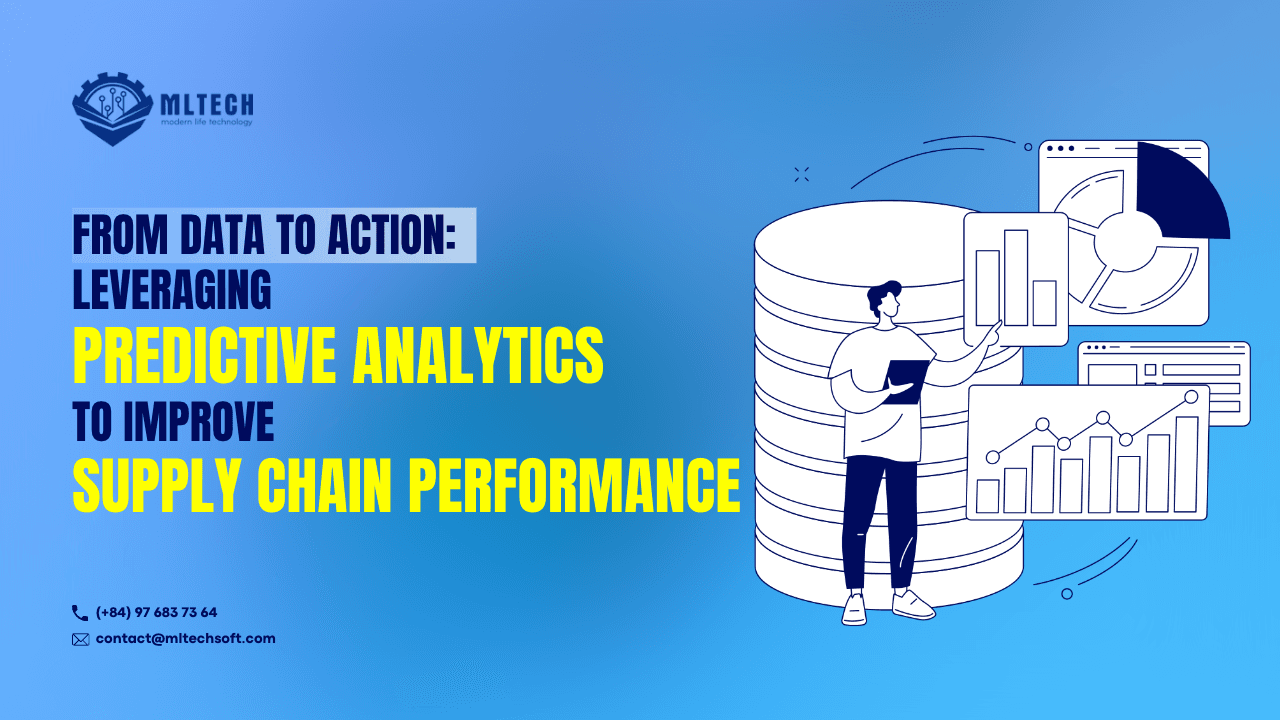
Big data, artificial intelligence, machine learning, data science, etc. are new words we hear a lot these days. These are all part of the new data revolution that is transforming businesses everywhere. By understanding data science, supply chain managers can better understand the impact on their business and how to improve performance and profitability.
We are currently in the midst of the Fourth Industrial Revolution, or Industry 4.0. This revolution is fueled by the availability of diverse enterprise data sources, empowering administrators to make decisions based on real-time data and predictive analytics. This revolution will transform the supply chain, empowering managers to make informed decisions using advanced analytics based on real-time data.
Predictive Analytics Technology
Today's organizations are inundated with data ranging from log files to images and videos, all of which reside in various data stores within the organization. To gain insight from this data, data scientists use deep learning and machine learning algorithms to find patterns and predict future events.
These statistical methods include logistic and linear regression models, neural networks, decision trees, and more. Some of these modeling techniques use initial predictive learning to obtain additional predictive insights.
Definition of predictive analytics
Predictive analytics is a branch of advanced analytics that uses historical data combined with statistical modeling, data mining techniques, and machine learning to predict future outcomes. Businesses use predictive analytics to find patterns in this data and identify risks and opportunities. Predictive analytics is often associated with big data and data science.
Data analysis consists of three pillars. These are the needs of entities that use models, data, and technology to study them, and the resulting actions and insights from using this type of analysis.
How are predictive analytics used in e-commerce?
Predictive analytics includes a combination of techniques and technologies such as AI, ML, and statistical analysis. From predicting stock market volatility to avoiding equipment failure, predictive analytics enables organizations to make informed decisions across a wide range of business areas.
In the e-commerce context, predictive analytics tools can be used to anticipate market needs, predict customer behavior, enable dynamic pricing, and detect fraud. It does this by finding relationships between various customer data points such as past purchases, demographics, social media sentiment, and web activity data.
At its core, predictive analytics enables continuous analysis of customer data, enabling advanced personalization capabilities. In today's increasingly competitive e-commerce environment, personalization is one of the key differentiators. A recent McKinsey study found that 76% of consumers are more likely to make a purchase when brands offer a personalized experience. Predictive analytics and big data e-commerce solutions enable businesses to create a unique online shopping experience for each customer.
Let's explore how companies are using predictive analytics to achieve this and discuss the nuances of implementation.
Benefits of Using Predictive Analytics to Forecast Demand
The benefits of predictive analytics in market research are numerous, and companies using this technique gain valuable insights that aid in their decision-making process. A Harvard Business Review study found that companies using predictive analytics are more likely to see increased sales and profitability.
Improved accuracy in predicting demand
Predictive analytics helps companies predict future trends and outcomes with a high level of accuracy. This allows brands to better plan their operations and resources to meet future needs. For example, a hotel chain can use predictive analytics to predict room occupancy and adjust staffing and inventory levels accordingly.
Better inventory management and reduction of stockouts
Out-of-stocks can be managed and often prevented by following good inventory management practices, including accurate forecasting of demand, reorder points, use of buffers and safety stocks, determination of inventory patterns, and use of inventory management software.
Increase customer satisfaction and loyalty
Customer satisfaction is important because it indicates whether your customer base likes your work. Research shows that higher satisfaction leads to higher customer retention, a higher lifetime value, and a stronger brand reputation. Low customer satisfaction is also important.
How do businesses use predictive analytics to forecast demand?
Demand forecasting is the area of predictive analytics that seeks to understand and forecast customer demand to optimize supply decisions by the supply chain and company management.
Collect data and identify trends
Trend analysis refers to collecting data from several different periods (sometimes called time series data analysis) before plotting the data on a horizontal line for validation. By comparing data over time, patterns can be identified and future events can be predicted.
Apply forecasting models
There are a wide variety of quantitative budget forecasting tools in common use, but this article will focus primarily on four methods:
(1) Linear, (2) Moving Average, (3) Simple Linear Regression, and (4) Multiple Linear Regression
Incorporate external factors
In addition to the methods described above, account managers can also use various forecasting tools to incorporate external factors and market trends into their customer forecasts. For example, sales forecasting software can help automate and streamline the forecasting process, while market research can provide insight and feedback from your target market.
Expert opinions, on the other hand, can offer a variety of perspectives and opinions, as well as best practices and case studies. All of these tools help account managers monitor and adjust forecasts in real-time to validate and improve customer forecasts.
Optimize Inventory Levels Using Predictive Analytics
Predictive inventory analysis tells inventory managers what is likely to happen in the future. Use these analyses to prepare and adjust to meet your requirements. For example, machine learning uses technology to learn from the past and predict the future.
Importance of optimizing inventory levels
By optimizing inventory, you can reduce working capital (by reducing unnecessary inventory buffers) and improve service levels. Ensure your customers get what they want, when they want it, without having to carry too much inventory. How can predictive analytics help determine optimal inventory levels?
Use statistical models to forecast future demand based on historical data. Predictive analytics helps inventory planners anticipate spikes in demand, identify potential supply chain disruptions, and adjust inventory levels accordingly.
Key Performance Indicators (KPIs) for Predictive Analytics in E-commerce
A KPI is a way to measure the leading (predictive and prescriptive analytics) and lagging (descriptive analytics) effectiveness of a unit of measure and progress toward achieving its goals. Essentially, KPIs reflect a company's strategic objectives for achieving business outcomes.
Identifying KPIs for forecasting demand and optimizing inventory levels: Demand planning KPIs are designed to provide up-to-date information on activities essential to planning. Some demand planning KPIs directly measure the results of demand planning work, such as: mean absolute percent error.
Monitor and analyze performance metrics: Metrics represent data in your system, while monitoring is the process of collecting, aggregating, and analyzing those values to improve your awareness of component properties and behavior.
Conclusion
Predictive analytics can take much of the guesswork out of planning and decision support processes. Unlike historical analytics, which only look at the past, predictive supply chain analytics enable supply chain leaders to anticipate and prepare for the future.
Get our blog
Want the latest and greatest from our blog straight to your inbox? Chunk us your details and get a sweet weekly email.
Read more in our blog
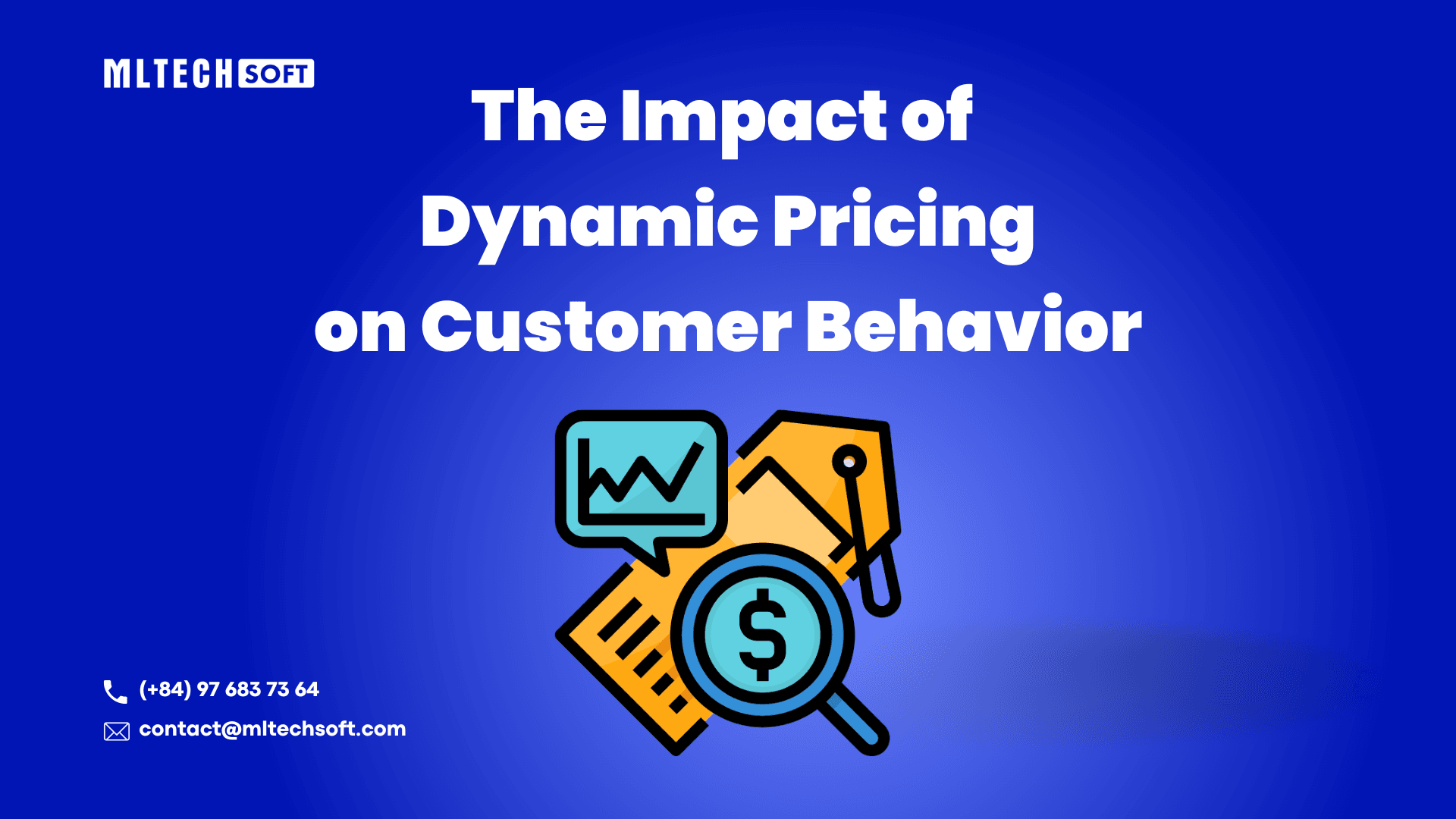
Project Management
The Impact of Dynamic Pricing on Customer BehaviorExplore the impact of dynamic pricing on customer behavior and learn how to implement effective pricing strategies.
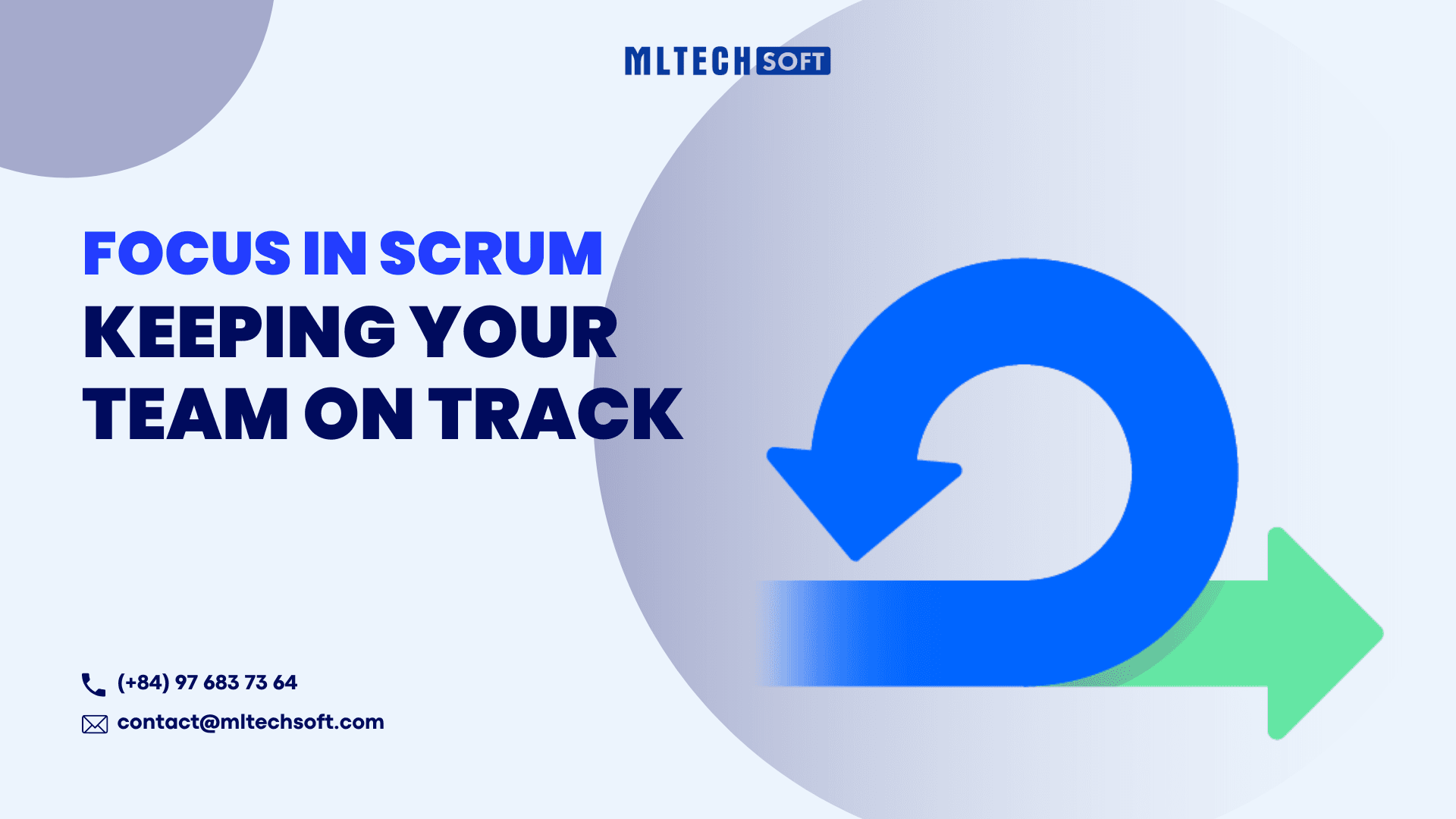
Project Management
Focus in Scrum: Keeping Your Team on TrackDiscover effective strategies to maintain focus within your Scrum team, overcome common challenges, and boost productivity.
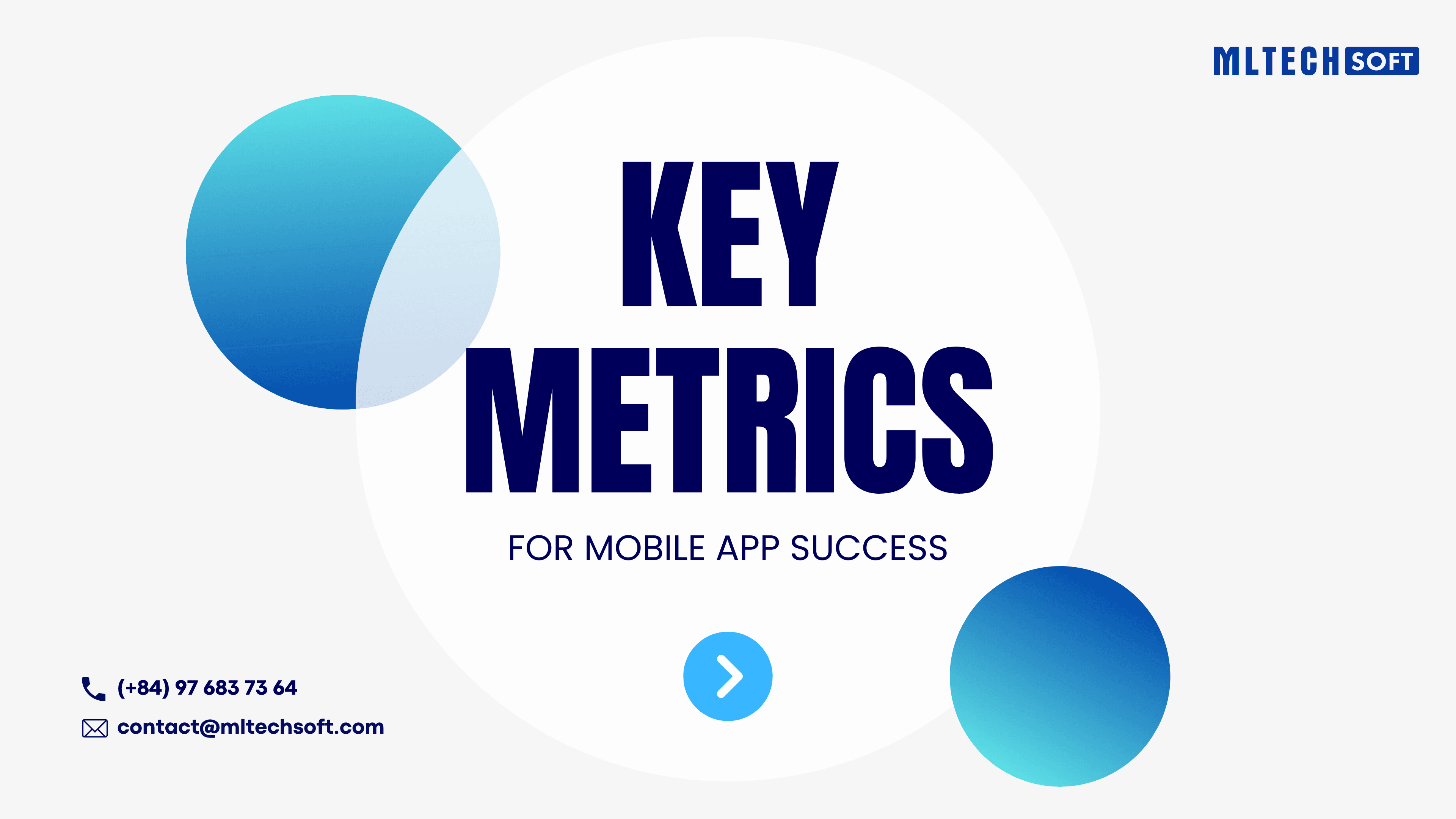
Project Management
Key Metrics for Mobile App SuccessDiscover the essential metrics for mobile app success, from user acquisition and engagement to monetization and performance.
MLTECH SOFT