Scroll down for more
Unlocking the Secrets of Customer Data: Enhancing Shopping Experiences with Personalization
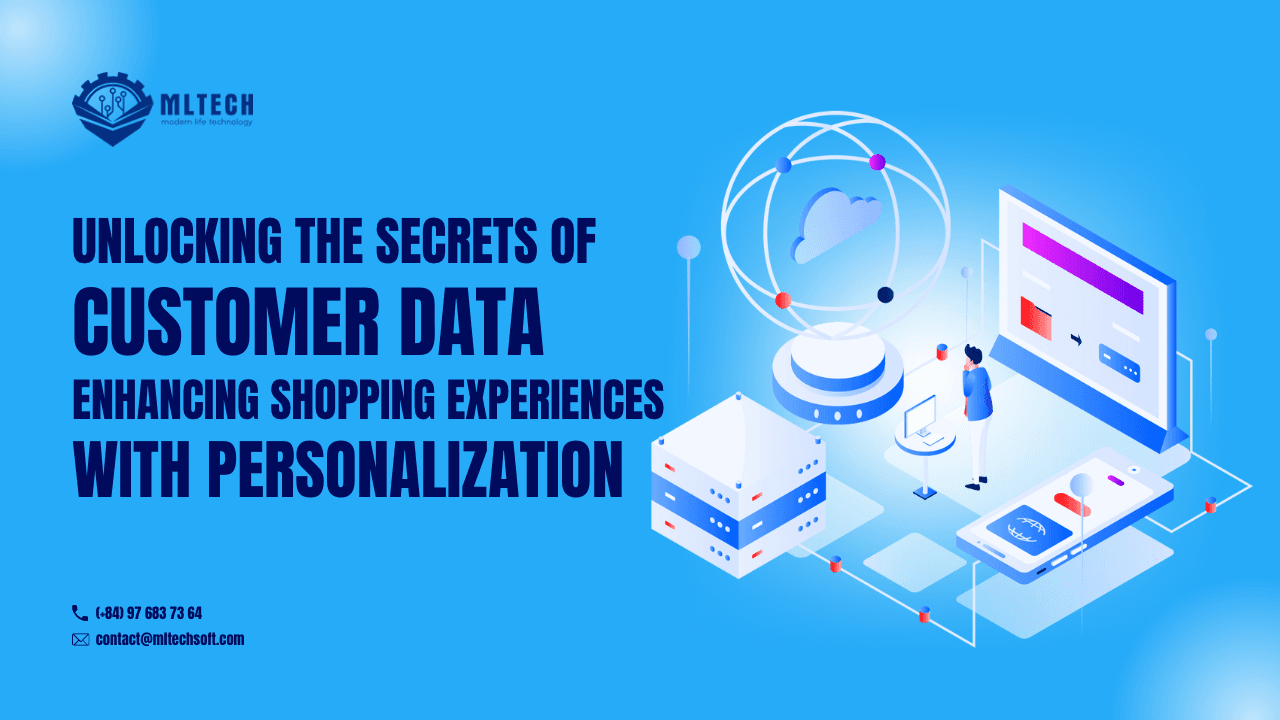
Personalization has come to be an extremely important aspect of the shopping experience. With the rise of online shopping, consumers are overwhelmed with an extensive range of choices, making it difficult to identify which items they desire.
The personalization of shopping experiences is an effective way for retailers to differentiate themselves in a competitive environment and provide a superior consumer experience.
This article is about unlocking the secret of customer data to boost customers' experiences in the shopping process through businesses’ personalization.
The Power of Personalization in E-commerce
Benefits of personalized product recommendations for customers and businesses
A personalized product recommendation is a common approach to buying strategy, so if businesses succeed in this way, they can bring many benefits both for customers and for themselves.
For customers, personalized recommendations can improve the shopping experience by helping them identify products that fit their interests, making shopping more efficient and pleasant. Besides, personalized recommendations offer suggestions tailored to their specific needs so they can save time while shopping.
Not only that, personalized suggestions will present customers with new products that they have not tried before. This improves their purchasing experience and potentially increases their loyalty.
Businesses will have more benefits than we think. Firstly, businesses can generate higher sales revenue and profits when customers make a purchase again through personalized recommendations.
Secondly, businesses can build stronger customer relationships as customers are pleased with the buying process, which leads to increased customer loyalty and retention.
Businesses can augment customer insights by referring to customer insights from personalized recommendations to improve their product offerings and marketing strategies. Furthermore, enterprises can differentiate and attract more new clients because their personalized recommendations offer a unique value proposition in the crowded market.
How data can be used to personalize product recommendations
Data is critical in the retail industry for assessing and delivering strategies and solutions to increase sales quality and the customer experience. It is at the heart of personalization and is used to tailor product recommendations in terms of:
-
Customer behavior: By analyzing data on customer behavior, such as their purchase history, browsing history, and search queries, retailers can understand their customers' preferences and interests and offer targeted product suggestions.
-
Demographic data: A retailer might recommend different products to younger people than older ones based on demographic data such as age, gender, location, and income level.
-
Social media data: By analyzing social media data such as likes, shares, and comments, retailers can get a sense of their customers' interests and preferences and offer targeted product suggestions.
-
Personalized marketing campaigns: A retailer might offer personalized promotions to customers who complete a survey or share their preferences and use this data to make personalized product recommendations.
There are numerous methods for utilizing and converting the raw data on hand into lively data with mutual aims. Retailers may improve consumer satisfaction and loyalty, increase sales income, and gain a competitive advantage by personalizing the shopping experience.
Collect and Analyze Customer Data
Types of data to collect for personalized product recommendations
Data is cleverly used for each aspect to improve personalized recommendations in business. So do businesses need to collect a lot of different information to get a good amount of data to help with this? That’s true in this case, businesses have to collect information from the:
-
Customer profile: This information covers basic demographics such as name, age, gender, location, and income level.
-
Purchase history: This information tells what the customer has previously purchased, how frequently they purchase, and how much they normally spend.
-
Browsing history: This information includes what the customer looked for on the retailer's website, how long they spent there, and whether or not they added things to their cart.
-
Search history: This information displays what they searched for on the retailer's website and what products they clicked on.
-
Social media data: This information comprises the customer's social media activity, such as likes, shares, and comments, as well as their social media profiles.
-
Feedback data: This data is derived from any feedback offered by the customer, such as product reviews.
-
Location data: This data comprises information about the customer's current location, which can be utilized to provide location-based recommendations or promotions.
-
Device data: This data comprises information about the customer's device used to visit the retailer's website, which can be used to provide device-specific recommendations or promotions.
The role of data analytics in identifying patterns and preferences
When faced with a large amount of data, businesses are often overwhelmed, and it is difficult to give an accurate result. Therefore, data analysis is very important for information processing and drawing an overall conclusion.
For recommendation personalization, data analysis helps businesses identify the factors and preferences of the target audience and then make decisions about suitable products, services, and strategies.
Data analytics can help identify patterns and preferences in these ways:
-
Segmentation: Data analytics can segregate client data into groups based on demographics, behaviors, and preferences. This assists firms in better understanding their clients and tailoring their offerings to match their needs.
-
Predictive modeling: Data analysis enables businesses to estimate future behavior, such as trends, based on historical data, and to make proactive decisions to satisfy client expectations.
-
Product optimization: Data analytics enables businesses to determine which items are the most popular and which features are most wanted by customers. This data can be utilized to improve products and better satisfy the needs of customers.
-
Marketing optimization: Organizations can use data analytics to determine which marketing channels and messaging are most effective in reaching their target audience. This can help businesses adjust their marketing efforts to increase client engagement and conversion rates.
How to segment customers based on data and behavior
As mentioned before, segmentation is one of the common ways to use data analytics to make personalized recommendations. It is the procedure of dividing customers into different groups based on specific characteristics or behaviors. Therefore, businesses can easily segment their on-hand data, and the steps below are to modify this process:
-
Identify the characteristics: Businesses can start by identifying the characteristics such as age, gender, income, or geographic location before segmenting their customers.
-
Collect the data: Collect the data needed to segment your customers, including customer purchase history, website analytics, or other relevant data.
-
Analyze the data: Use data analytics tools to analyze the data and identify patterns and trends that can be used to segment customers.
-
Group customers: Businesses may group customers based on the characteristics identified in step one. Test and refine: Based on the insights businesses gain from segmentation analysis, test and refine to match with purposes.
-
Use segmentation to personalize: This could involve tailoring your messaging or offers based on customer segmentation data to better meet the needs and preferences of each customer segment.
Develop Effective Recommendation Strategies
Effective strategies for recommendation can assist firms in increasing sales, improving customer loyalty, and improving the overall customer experience.
The importance of relevance in product recommendations
Relevancy is important in product recommendations since it ensures that the products offered are valuable and useful to the customer. In particular, relevancy increases customer engagement through personalized items matching their interests and needs.
Furthermore, when clients receive relevant recommendations, they are more likely to have a pleasant experience with the business, which leads to improved customer loyalty and repeat business. As a result, businesses might create more revenue and earnings than they did previously.
In some circumstances, businesses can boost customer retention and attract new consumers by making relevant recommendations. Because they see businesses offering items that are relevant to their needs and meet those needs.
Strategies for using data to improve product recommendations
Data has an essential role in identifying patterns and preferences, which contribute to building product recommendations. That’s why retail businesses must create relevant strategies to improve product recommendations by using on-hand data.
Here are some suggested strategies businesses can apply:
-
Collect and analyze customer data: Collect and analyze consumer data to uncover patterns and trends that can be used to inform product suggestions.
-
Use machine learning algorithms: Machine learning algorithms can aid in the identification of patterns and connections between various items and client behaviors.
-
Integrate product data: Integrate product data into the suggested purchase engine, such as product descriptions, features, and specifications, to increase suggestion precision.
-
Consider different types of recommendations: Provide an array of recommendations based on a customer's status during the purchasing process, such as watch and bought. Customers may discover new items and services that they would not have discovered otherwise.
-
Test and refine: Put different recommendation tactics to the test and improve them based on customer feedback and sales statistics. This can assist you in determining which techniques are most beneficial for your company.
-
Use A/B testing: A/B testing can be used to compare different suggestion tactics and determine which one is more effective at driving sales.
-
Use real-time data: Use real-time data to present clients with up-to-date recommendations based on their current activity and interests.
The Role of artificial intelligence and machine learning in product recommendations
Artificial intelligence (AI) and Machine Learning (ML) make significant contributions in all sectors, including retail. Both technological tools are crucial for product suggestions since they allow businesses to deliver individualized and relevant recommendations to their clients. Al and ML can do more than we think, involving:
-
Different types of client information can be analyzed by AI and ML algorithms to deliver customized suggestions based on their interests and needs. Then it can forecast what clients are likely to buy.
-
AI and ML algorithms can provide real-time recommendations based on a customer's current behavior, allowing businesses to monitor and gain insight into their consumers' purchasing habits.
-
AI and machine learning algorithms can use contextual information, such as the customer's location or weather conditions, to deliver more appropriate recommendations.
-
AI and ML algorithms can utilize collaborative filtering approaches to identify comparable customers and recommend products based on what they have purchased in the past.
-
AI and ML algorithms can deliver increasingly accurate recommendations that help boost sales and improve the customer experience by evaluating and learning from customer data over time.
Implement and Test Personalized Recommendations
One of the suggested tactics in personalized recommendation is to implement and test personalized recommendations, which aim to evaluate what is effective to continue.
How to implement personalized product recommendations on your website
There are various processes involved in implementing personalized product recommendations on your website. They are as follows:
-
Select a recommendation tool: Collaborative filtering, content-based filtering, and hybrid filtering are three types of recommendation technologies. Choose the greatest match for your company's goal.
-
Gather client information: Gather information about client behavior and preferences to help inform product suggestions.
-
Prepare your data as follows: Prepare your data so that it can be used in the recommendation engine. This may entail cleaning and formatting data to ensure that it is in a compliant format.
-
Choose your recommendation strategy: Define your recommendation approach, including what types of recommendations you will make and how you will present them.
-
Install the recommendation engine: Incorporate a recommendation engine into your website. It may be necessary to integrate it with your e-commerce platform or content management system.
-
Test the recommendations: Monitor customer behavior and track the impact of the advice on sales and engagement metrics to put the recommendations to the test.
-
Refine the suggestions: Businesses can adjust suggestions based on consumer feedback and performance data to ensure they remain relevant and effective.
-
Monitor and optimize: Monitor the recommendation engine's effectiveness and optimize it over time to provide the most personalized recommendations.
Businesses may improve consumer engagement, drive revenue, and improve the customer experience by making relevant and personalized recommendations.
The benefits of A/B testing and experimentation in improving recommendations
A/B experimentation and testing are techniques for comparing two versions of something (such as a web page, an email, or a recommendation system) to see which one performs better. Therefore, businesses consider it a powerful technique for improving recommendations.
Some exceptional benefits that A/B testing and experimentation bring are:
-
Improved relevance: By testing alternative suggestion tactics and analyzing consumer interaction, A/B testing and experimentation can assist find which product recommendations are most relevant to customers.
-
Increased conversions: Businesses can optimize their suggestions to create more sales and revenue by trying alternative recommendation tactics and analyzing the impact on conversion rates.
-
Better customer experience: By giving highly relevant and tailored recommendations, organizations can improve customer happiness and experience.
-
Deeper data insights: By examining experiment outcomes, organizations can obtain a better knowledge of their customer's requirements and preferences, which can then be used to guide future recommendations and product development.
-
Continuous improvement: Businesses can ensure that their recommendations remain relevant and successful by testing and refining them as customer behavior and preferences change.
How to measure the success of personalized recommendations
The number will be the true proof of accomplishment, as will the success of individualized recommendations. Key performance indicators (KPIs) can be used by businesses to demonstrate the impact of recommendations on business outcomes.
Here are some KPIs to consider:
-
CTR: Calculate the percentage of customers who click on recommended products. The greater the CTR, the more engaged the customers.
-
Conversion rate: The percentage of customers that buy a product after clicking on a recommendation. The higher the conversion rate, the more engaged the customers.
-
Average order value (AOV): Calculate the average value of orders containing recommended products. The greater the AOV, the more effective the recommendations are at promoting higher-value products.
-
Customer lifetime value (CLV): Estimate the worth of customers who use recommended items over time. The greater the CLV, the more effective the advice in fostering long-term client loyalty.
-
ROI: Calculate the revenue generated by recommended products vs the cost of deploying and maintaining the recommendation engine. A positive ROI shows that the recommendations are a worthwhile company investment.
-
Customer feedback: Gather feedback from customers about their personalized recommendations experience. This can provide useful insights into what customers find useful and interesting.
Case Studies: Examples of Effective Personalization Strategies
Examples of businesses that have successfully used data to personalize product recommendations
Indeed, many successful organizations have used data to tailor suggestions to their respective professions and industries. Typically, we must state:
-
Amazon: Amazon's recommendation engine provides individualized product recommendations based on consumer data such as search history and purchase history. They also employ machine learning algorithms to improve their recommendations over time.
-
Netflix: Netflix's recommendation engine provides customized movie and TV show recommendations based on customer data such as viewing history and ratings. They also utilize machine learning algorithms to enhance their recommendations over time and even develop unique content depending on client preferences.
-
Spotify: Spotify's recommendation system provides individualized music recommendations based on consumer data such as listening and search history. They also utilize machine learning algorithms to improve their recommendations over time and even build personalized playlists for consumers.
An analysis of the strategies and tactics used in these examples
These examples have one thing in common: using similar strategies and tactics in personalized products involving: Collecting and analyzing customer data: All of the businesses mentioned collect and analyze customer data to inform their recommendation strategies. This data includes things like purchase history, search history, and viewing history.
-
Machine learning algorithms: Machine learning algorithms are used by all of the organizations mentioned to continuously enhance their recommendation tactics. These algorithms investigate client data to find patterns and preferences before generating customized recommendations.
-
Personalization at scale: All of the companies mentioned are capable of providing customized recommendations on a large scale. This is accomplished by utilizing innovative technology and algorithms capable of processing massive amounts of data rapidly and accurately.
-
Testing and experimentation: Many of these businesses update their suggestion tactics through A/B testing and experimentation.
-
Providing a seamless customer experience: All of the firms mentioned providing a consistent customer experience that allows clients to easily engage with customized recommendations.
-
Investing in technology and talent: To support their tailored recommendation techniques, all of these companies have made major investments in technology and expertise, hired data scientists and machine learning professionals, as well as invested in modern technological platforms.
Lessons learned from these case studies
Through the examples above, we can glean some of the key lessons about using data to personalize product recommendations:
-
Collecting and analyzing customer data is crucial: Because it helps businesses have a clear segmentation so they will know to prioritize data collection and analysis to provide effective personalized recommendations.
-
Machine learning is key to success: These algorithms evaluate client data to find patterns and preferences before making recommendations based on that knowledge. The more they invest in technology, the more benefits they receive.
-
Personalization at scale is possible: Thanks to the use of advanced technology and algorithms, businesses can process large amounts of data quickly and accurately.
-
Testing and experimentation are critical: They can improve their recommendations by testing alternative tactics and monitoring client involvement. To consistently enhance their personalized recommendation techniques, businesses must prioritize testing and experimentation.
-
Providing a seamless customer experience is important: All of the organizations described in the case studies provide a smooth customer experience that allows customers to easily engage with personalized recommendations, hence driving engagement and customer satisfaction.
Overall, the case studies show that organizations can utilize data to successfully give individualized product suggestions at scale. Businesses may create stronger engagement, more revenue, and enhanced customer satisfaction by implementing the suggested strategies above.
The Future of Personalized Product Recommendations
The future of personalized product recommendations is bright. For businesses, as they continue to collect more data and invest in machine learning and AI technology, their ability to provide highly personalized recommendations at scale will only improve.
And customers are always increasingly expecting personalized experiences, so businesses that can provide tailored recommendations will have a competitive advantage. This is especially true for e-commerce businesses, where personalized recommendations can lead to higher conversion rates and increased customer loyalty.
However, personalized product recommendations mainly relate to data, so they may pose a potential risk to data privacy and security. That's why businesses must focus on protecting data as tightly as possible.
Content
Get our blog
Want the latest and greatest from our blog straight to your inbox? Chunk us your details and get a sweet weekly email.
Read more in our blog
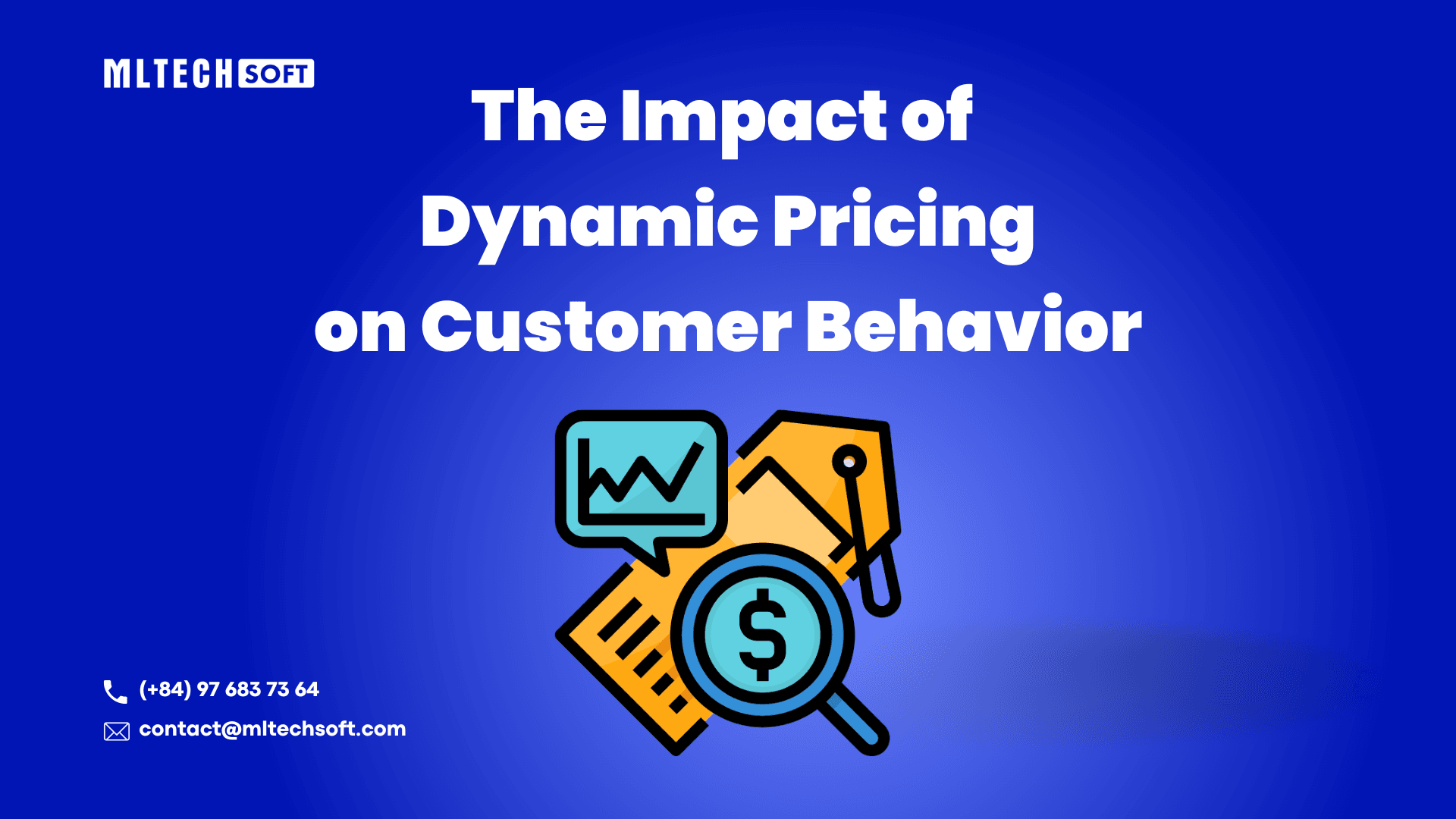
Project Management
The Impact of Dynamic Pricing on Customer BehaviorExplore the impact of dynamic pricing on customer behavior and learn how to implement effective pricing strategies.
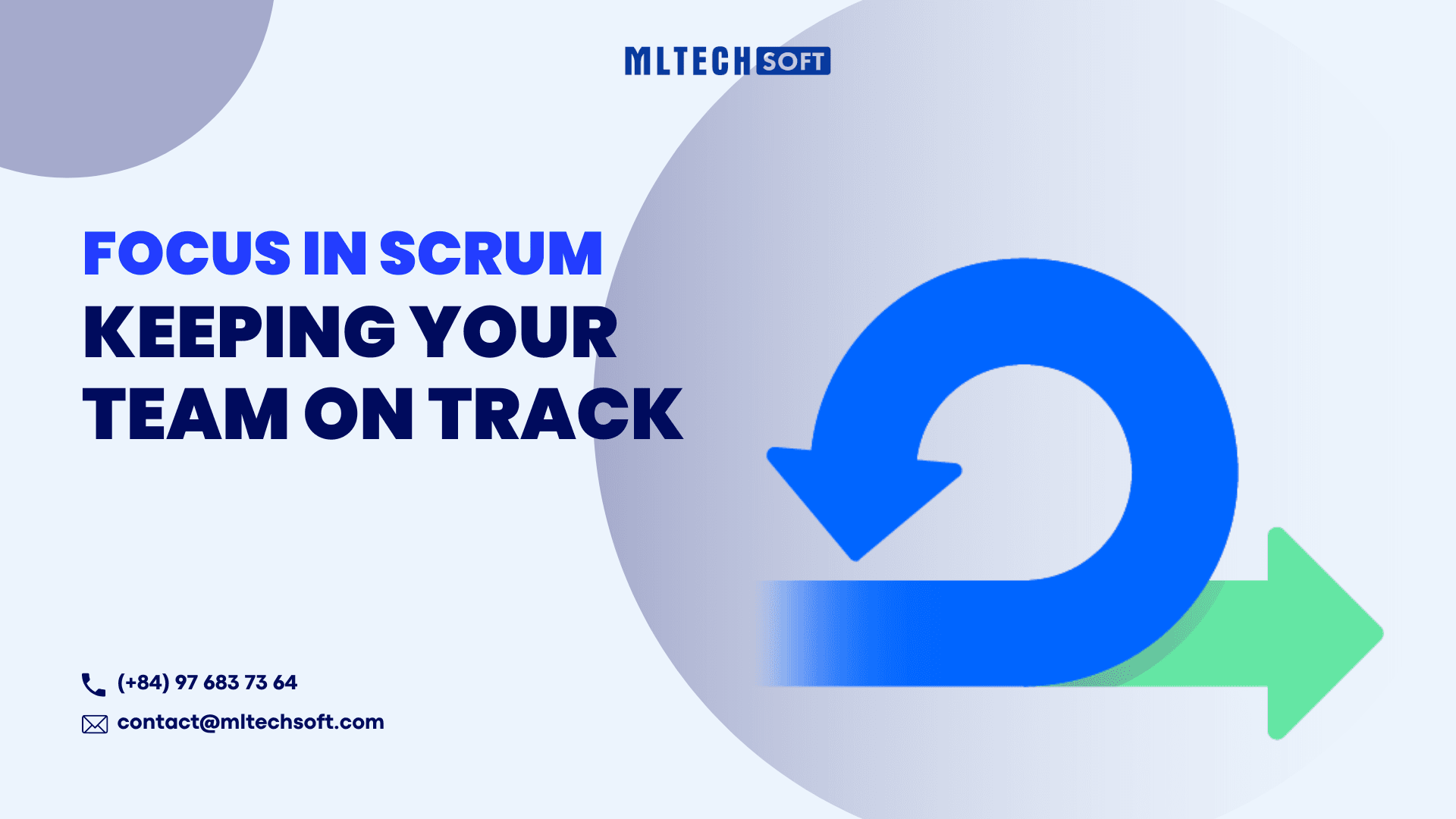
Project Management
Focus in Scrum: Keeping Your Team on TrackDiscover effective strategies to maintain focus within your Scrum team, overcome common challenges, and boost productivity.
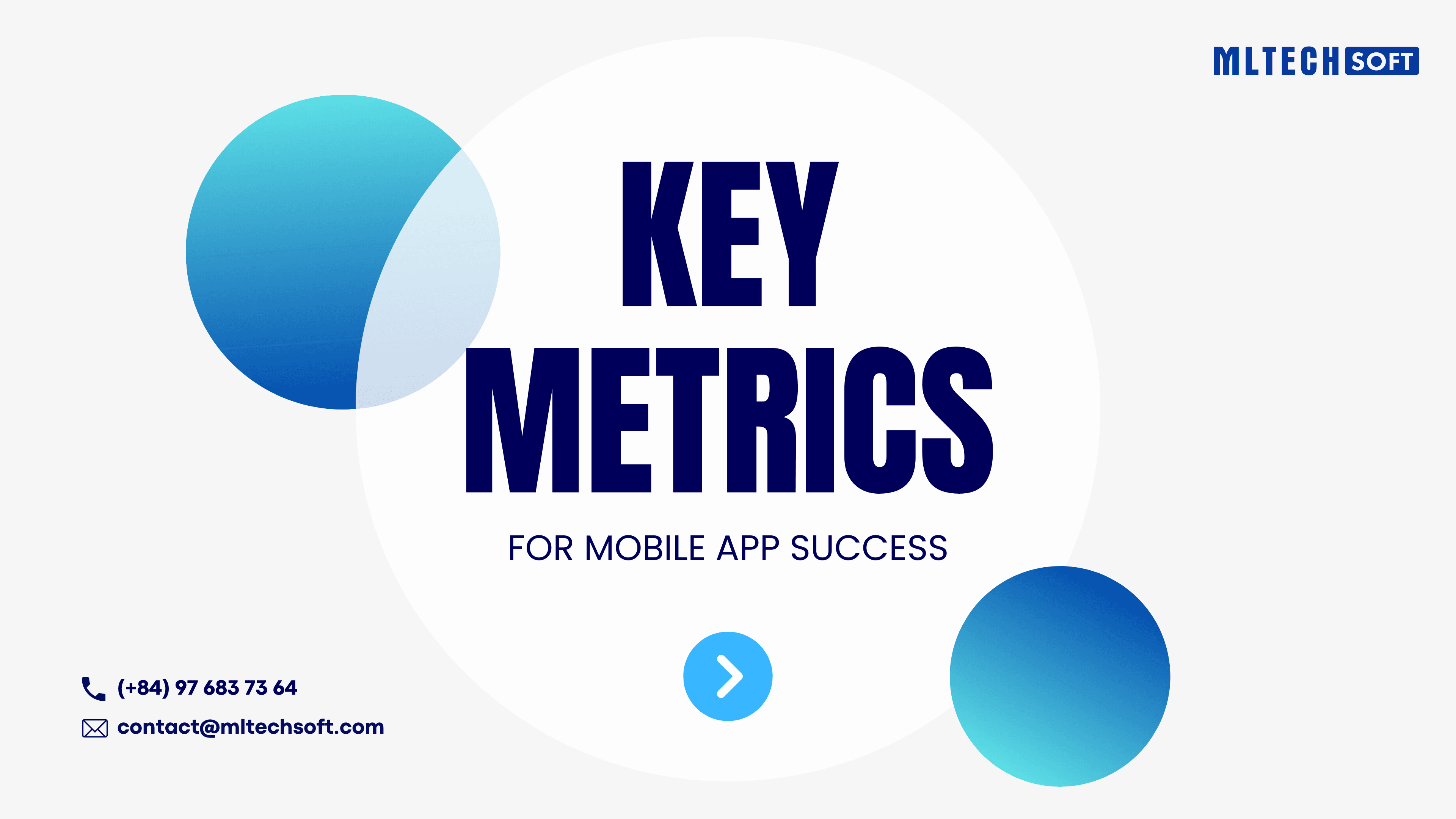
Project Management
Key Metrics for Mobile App SuccessDiscover the essential metrics for mobile app success, from user acquisition and engagement to monetization and performance.
MLTECH SOFT