Scroll down for more
Big Data Analytics: Unlocking Insights for Smarter Inventory Management and Optimization
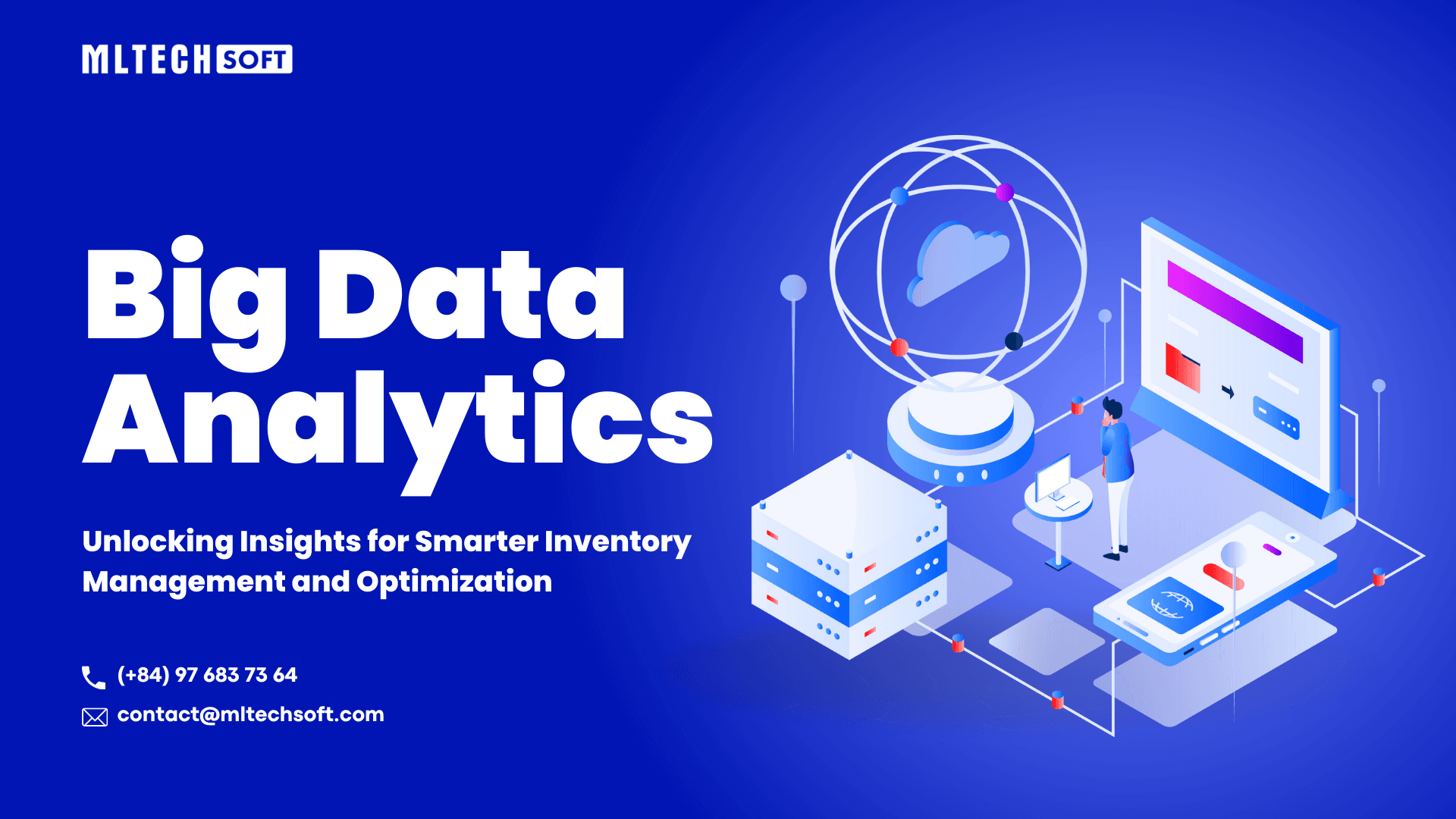
Get real-time information about supply chain operations and warehouse management flows with the help of big data analytics. This helps identify bottlenecks, improve inventory planning efficiency, and avoid unnecessary waste of time, money, storage space, and resources.
1. Understanding Big Data Analytics in Inventory Management
Big data helps businesses track inventory levels in real time. This reduces the risk of out-of-stock or overstock. By analyzing data from sensors, RFID tags, and other sources, businesses can understand inventory levels and make data-driven decisions about when and where to replenish inventory.
1.1. Big data analytics in the context of inventory management
Inventory analytics tracks metrics that measure physical product movement and performance. Continuous inventory valuation gives you the insight you need to optimize inventory availability to meet demand while minimizing inventory costs.
1.2. Key components of big data analytics: volume, velocity, variety, and veracity
Volume: You may have heard, once or twice, that big data is nothing more than business intelligence, even though it is very large in form. However, more data is not synonymo us with that. Big data obviously requires a certain amount of data, but just because it's big data doesn't mean you're working in that space.
Variety: We can now make decisions based on prescriptive data obtained in this way. Thanks to this technology, every action taken by customers, competitors, suppliers, etc. generates prescriptive information ranging from structured and manageable data to unstructured information that is difficult to use for decision making
Veracity: This V relates to both data quality and availability. When it comes to traditional business analytics, data sources become more quantitative and diverse. However, it gives organizations greater control over them and makes them more reliable.
Velocity: Without the sheer volume of information being processed, and, more importantly, the speed with which it can be processed, diversity and veracity will become less important and under greater pressure in big data efforts. It is quite possible that you will be exposed. Information must be generated and managed.
1.3. Role of data sources and technologies in collecting and analyzing large-scale inventory data
Big data analytics is important because it enables organizations to leverage large amounts of data from multiple sources and in multiple formats to identify opportunities and threats, enabling them to act quickly and improve their bottom lines. Some characteristics of big data are volume, variety, speed, and variability. Benefits of big data include improved decision-making, better customer service, and increased operational efficiency.
Big data analytics is the process used to uncover meaningful insights such as hidden patterns, unknown relationships, market trends, and customer preferences. Among other things, it can be used to improve decision-making and prevent fraud.
1.4. Benefits of Big Data Analytics in Inventory Management
Big data analytics combines data and quantitative techniques to enable better decision making for all supply chain operations. This extends the data set for analysis beyond the typical internal data stored in ERP systems.
Improved demand forecasting and inventory planning. Inventory forecasting tools help you automate reordering, anticipate labor requirements, and account for changes in order volume, making it easier to understand what's coming and reducing inventory costs. This saves time and manpower for warehouse management and staff overall.
Enhanced inventory visibility and real-time monitoring. Real-time inventory management involves implementing technology that can track, view, and manage inventory levels on-demand throughout the e-commerce supply chain.
Identification of inventory patterns, trends, and anomalies. Identifying patterns and trends is a technique analysts use to study supply and demand for publicly traded assets. A trend is the general direction of price over a period of time.
Optimized inventory levels and reduced stockouts. Inventory optimization is a set of best practices for businesses looking to reduce capital usage while maintaining salable inventory. This is an important part of inventory management, considering fluctuations in demand and supply.
Cost savings through better inventory control and procurement strategies. Cost-saving strategies include better price negotiations with suppliers, standardization of procurement processes, the use of e-procurement tools, consolidation of purchases, and improved supplier relationships. Reduce procurement costs by better negotiating prices with suppliers.
2. Key Applications of Big Data Analytics in Inventory Management
2.1. Demand forecasting and predictive analytics
Predictive analytics uses systematic computational analysis (analytics) of data or statistics to find out why, rather than just predicting the demand itself. Demand planning only creates an estimate of demand - predictive analytics creates a future "if" assessment
2.2. Real-time inventory tracking and replenishment
Real-time inventory management is an automated process that uses software to record sales and purchases. Having a complete view of what is happening in inventory enables organizations to respond quickly to the needs of their supply chain.
2.3. Sales and inventory data analysis for market insights
Historical sales data provides businesses with insight into when, where, what, and how consumers make purchasing decisions. These insights should be at the core of any effective sales organization.
2.4. Supply chain optimization and risk management
Improve the efficiency and performance of your delivery network to give your customers what they want, when they want it, and where they want it, while making your business more profitable and sustainable.
3. Implementing Big Data Analytics in Inventory Management
Collecting and integrating relevant inventory data from various sources. Data integration is the process of combining data from various sources into a single, unified view. Integration begins with the ingestion process and includes steps such as cleansing, ETL mapping, and transformation.
Utilizing data visualization and reporting tools for actionable insights. Data visualization is the creation of a visual representation of data. These representations clearly communicate insights from data through charts and graphs. In the spirit of business intelligence (BI), these visualizations help users make better data-driven decisions.
3.1. Applying statistical models and machine learning algorithms for analysis
Predictive statistical analysis is a type of statistical analysis that analyzes data to infer past trends and use them to predict future events. Perform statistical analysis of data using machine learning algorithms, data mining, data modeling, and artificial intelligence.
3.2. Overcoming challenges of data quality, data security, and privacy
a. Be aware of insider threats
Identify risky actors and respond quickly to suspicious activity. Monitor security systems and respond to suspicious or disruptive behavior according to incident response policies. Monitor and control remote access to your organization's infrastructure.
b. Encrypt all your devices
Encryption is the process of encrypting all user data on an Android device using a symmetric encryption key. When a device is encrypted, all user-generated data is automatically encrypted before being transferred to disk, and all read operations automatically decrypt data before returning it to the calling process
3.3. Building a data-driven culture and fostering collaboration across departments
Here are some steps you can take to build a data-driven culture in your workplace.
Invest in your data infrastructure. For all types of businesses, the benefits of using data strategically or prioritizing a good data infrastructure include reduced costs, reduced time, streamlined product development and allocation, and better information, which includes decision-making based on
Encourage exhaustive data collection. Of all quantitative data collection methods, surveys and questionnaires are among the simplest and most effective. Since surveys and questionnaires can be applied to both quantitative and qualitative research, many graduate students doing doctoral research use this method.
Improve data quality. From a financial perspective, maintaining high data quality can help organizations reduce the costs of identifying and correcting bad data in their systems. It also helps organizations avoid operational errors and business process failures that can lead to increased operating costs and reduced revenue.
4. Future Trends and Opportunities in Big Data Analytics for Inventory Management
4.1. Evolution of big data analytics technologies and techniques
Big data technology has come a long way since the early days of data warehousing. The advent of Hadoop, NoSQL databases, cloud computing, machine learning, data streaming, and edge computing has revolutionized how big data is stored, processed, and analyzed.
4.2. Role of artificial intelligence (AI) and machine learning (ML) in advanced inventory analytics
Machine learning has emerged as a subfield of artificial intelligence, specifically using algorithms and statistical models to enable computers to learn and make predictions. When it comes to inventory management, machine learning is revolutionizing how businesses track and manage inventory.
4.3. Predictive analytics for supply chain risk management and proactive decision-making
Using predictive analytics, businesses can identify optimal inventory levels to meet demand while minimizing inventories. Supply chain managers can use predictive analytics with sophisticated models to determine detailed inventory needs by region, location, and use.
Conclusion
By efficiently automating inventory management, you can ensure that popular items are always in stock to meet customer demand. Accurate information about fulfillment plans improves the customer experience and helps build brand loyalty.
Get our blog
Want the latest and greatest from our blog straight to your inbox? Chunk us your details and get a sweet weekly email.
Read more in our blog
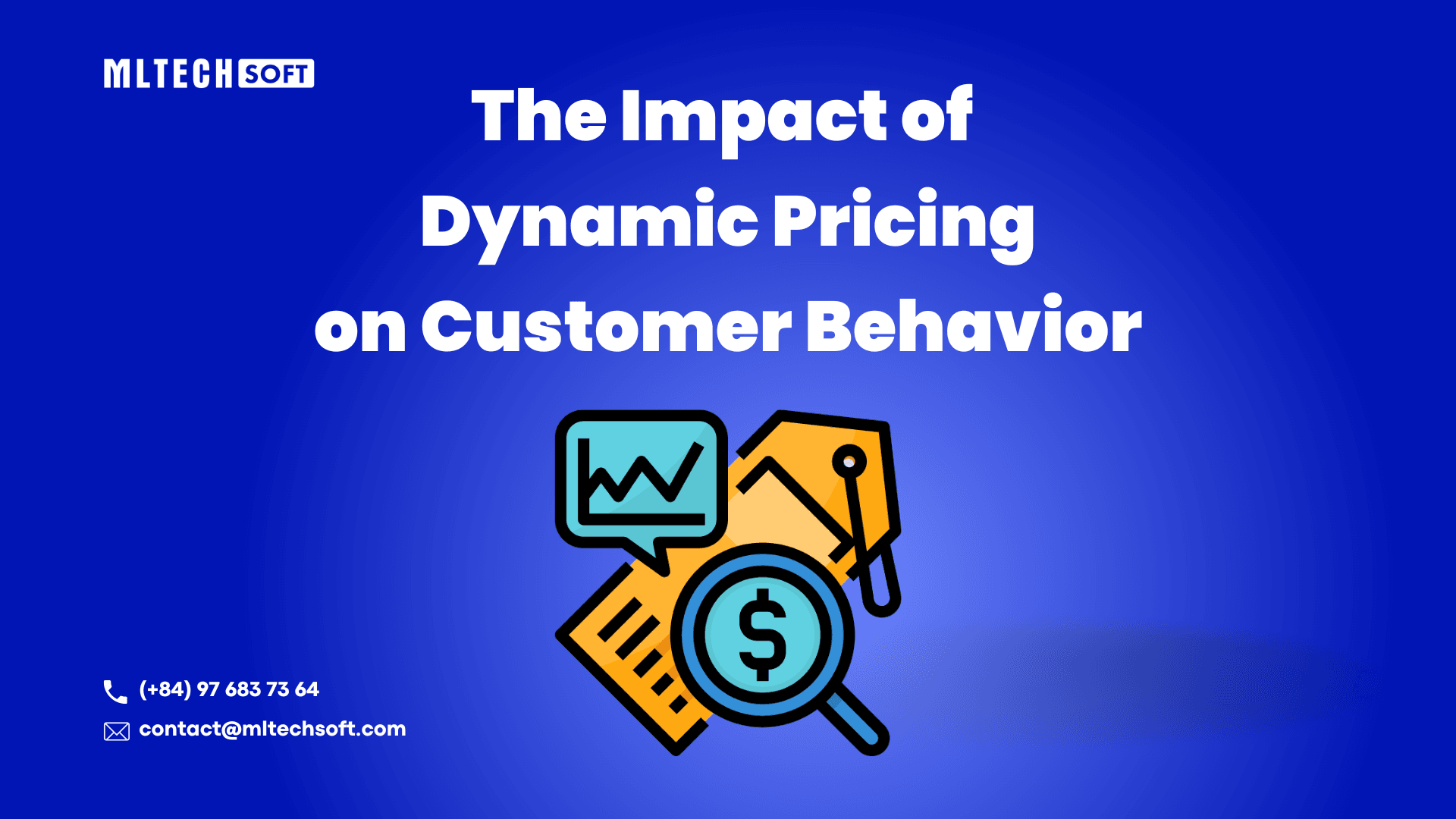
Project Management
The Impact of Dynamic Pricing on Customer BehaviorExplore the impact of dynamic pricing on customer behavior and learn how to implement effective pricing strategies.
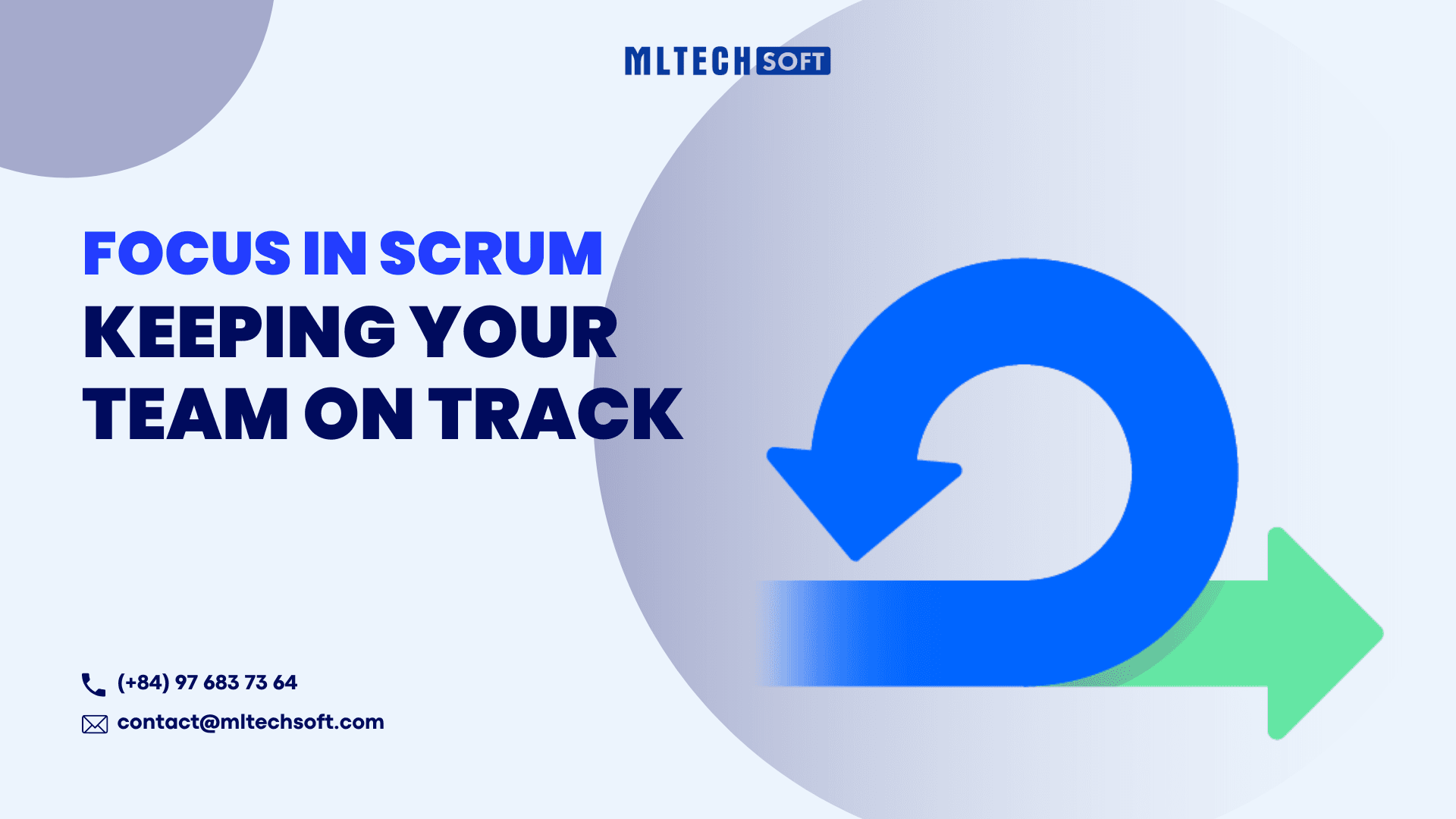
Project Management
Focus in Scrum: Keeping Your Team on TrackDiscover effective strategies to maintain focus within your Scrum team, overcome common challenges, and boost productivity.
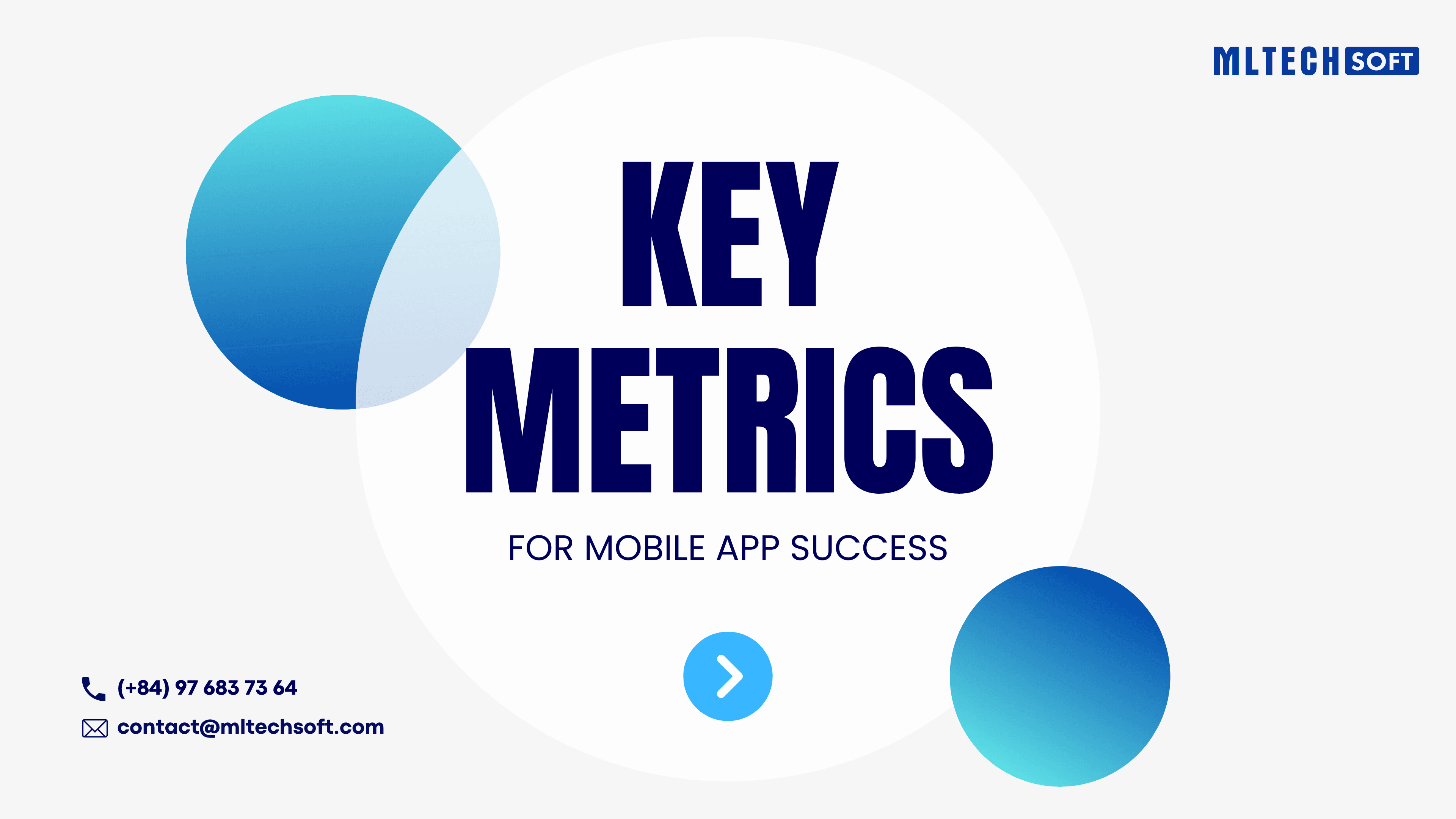
Project Management
Key Metrics for Mobile App SuccessDiscover the essential metrics for mobile app success, from user acquisition and engagement to monetization and performance.
MLTECH SOFT